Is ChatGPT Enough? Why Businesses Seek Enterprise AI Search
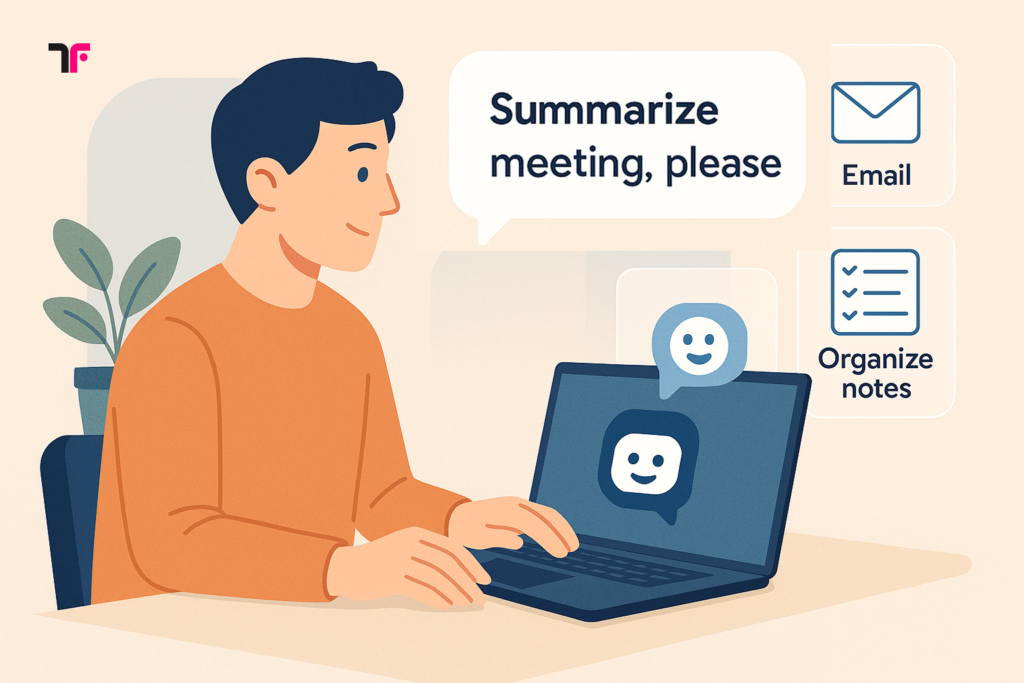
“Draft an email.” “Summarize a meeting.” “Organize notes.” We live in an era where professionals type commands at their desks rather than ask colleagues. Generative AI like ChatGPT, Perplexity, and Grok have shifted communication from people to machines, replacing Google searches and even offering near-expert-level responses. That’s not all. With just a simple prompt, Generative AI can extract insights, tailor content to different audiences, and draft strategic plans in seconds. You might think, “If it can do all this, can one person handle a hundred tasks?” And are you actually handling the work of a hundred people now? Despite Using ChatGPT, We Still Spend 2.5 Hours a Day Searching According to IDC, office workers spend 2.5 hours a day searching for information, which adds up to nearly 30% of their workday and 650 hours a year. The reason is clear. we use too many productivity tools at work, scattering our data across multiple platforms like Notion, Google Drive, Slack, Figma, and Dropbox. The more tools we have, the more fragmented our data becomes, making searches increasingly complex. So, why can’t ChatGPT solve this? The Weaknesses of Generative AI: Questions ChatGPT Misses “What was our team’s scope during the new project meeting?” “How much of our marketing budget remains this quarter?” “Has Legal reviewed the new advertising contract?” ChatGPT can’t really answer these questions. Why? Because Generative AI like ChatGPT doesn’t know your company’s data — and it doesn’t have permission to access it either. Instead, it’ll probably just throw out some generic advice or make something up. And it’ll sound very confident while doing it. Generative AI’s Structural Limitations No Access to Internal Data Generative AI tools like ChatGPT are trained on publicly available web data. It cannot access your company’s internal tools like ERP, CRM, Google Drive, Slack threads, meeting notes, or contracts, meaning it can’t provide accurate answers based on real data. No Understanding of Work Context Generative AI lacks awareness of why a document was created, who authored it, what project it supports, and how it fits into broader workflows. Without this understanding, it cannot link related documents, track project progress, or reflect the nuances of internal business processes, creating significant limitations. Security and Privacy Vulnerabilities Most generative AI services, including ChatGPT, run on cloud-based systems where users enter prompts and receive answers in real time. This setup is convenient, but not without risk. In March 2023, a bug caused ChatGPT to show other users’ chat titles and billing details. In 2024, Italy fined OpenAI €15 million for collecting personal data without clear consent. These cases show that generative AI must handle user data with stronger privacy protections. Hallucinations Risks in Business A hallucination in generative AI refers to a response that is entirely or partially made up, yet presented as if it were true. These outputs often sound confident and credible, which makes them especially dangerous in business settings. For example, a support chatbot giving incorrect information may harm a company’s reputation and reduce customer trust. Faulty outputs can also mislead decision-makers, leading to financial loss or strategic mistakes. Hallucinations: Real-World Cases OpenAI Case In 2024, OpenAI introduced its latest models, o3 and o4-mini, aiming to enhance reasoning capabilities. However, research found that these models exhibited a higher rate of hallucinations compared to their predecessors. (Source: OpenAI’s new reasoning AI models hallucinate more) Google Bard Case In 2023, Google’s Bard AI incorrectly claimed the James Webb Space Telescope (JWST) captured the first image of a planet outside our solar system—actually captured by the Very Large Telescope (VLT) in 2004.This error caused a 7.7% drop in Alphabet’s stock price, wiping out $10 billion in market cap. (Source: Best Ways to Prevent Generative AI Hallucinations in 2025) Even with better prompting, hallucinations remain an unresolved challenge in 2025. Why Do Hallucinations Occur? Hallucinations in Generative AI occur because they predict the most statistically probable sequence of words based on the patterns they learned during training. When the Generative AI like Chat GPT encounters gaps in its knowledge or ambiguous prompts, it fills in the missing information with plausible-sounding, but often inaccurate, content. This happens because Generative AI does not verify facts against an external source during response generation—they are a byproduct of how probabilistic language models. Enterprise AI Search Controls Hallucination with RAG Controlling hallucinations through RAG is a key reason why businesses choose Enterprise AI Search over generative AI. This capability clearly distinguishes the two. RAG (Retrieval-Augmented Generation) is a method that improves AI reliability by combining search before generation. Instead of relying solely on learned patterns or internet search, it retrieves relevant information from internal company data before generating a response. This approach reduces the risk of hallucinations and helps Enterprise AI Search deliver accurate, context-aware answers based on real facts, instead of pretending to know. Why Businesses Need Enterprise AI Search 📚Verified Data Sources In business, trust depends on evidence. We constantly ask what the source is and whether there is proof. Generative AI relies on public web content, which may include information that is outdated, biased, or even manipulated. Without knowing who wrote it or how accurate it is, referencing such data can pose real risks for businesses. Enterprise AI Search avoids this by focusing only on company-owned data. It connects directly to internal systems such as ERP, CRM, Google Drive, Notion, and Slack threads to retrieve and verify real-time documents and communications. Because it uses data already managed and trusted by the company, it delivers reliable answers aligned with real business operations. 🤖 The Purposes: Generative AI vs. Enterprise AI Search Generative AI: Designed for CREATING content. Enterprise AI Search: Built to retrieve, integrate, and ACCELERATE DECISION-MAKING based on verified company data. As Gartner (2023) defines it, Enterprise AI Search enhances organizational productivity and operational efficiency, not by generating random content, but by helping employees find the right information faster. This shows that businesses need AI tools purpose-built for their real operational needs. 🧠 Context-Aware AI Assistance in the Workplace Enterprise AI understands the flow of a project by tracing the history